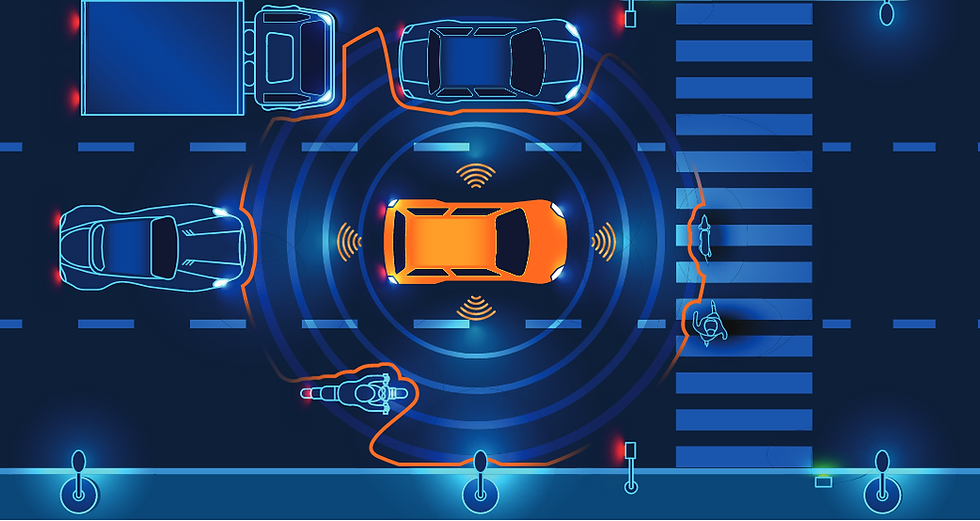
To address the operational and safety concerns associated with roadway traffic, various conventional solutions, including capacity expansion, have been implemented. However, due to a lack of space and funding resources, conventional solutions cannot fully address the traffic issues anymore (Ajitha et al., 2015). Therefore, the focus has been shifting towards improving the performance of the current infrastructure by using operational tools and implementing intelligent transportation system (ITS) technologies. Among those, autonomous vehicles (AVs) have been considered as an effective solution. Hence, the safety and operational benefits of AVs on various roadway components should be evaluated comprehensively, before implementing them on the current roadway infrastructure.
The World Health Organization (WHO) reported 1.35 million fatalities due to roadway crashes in 2018. In addition, roadway crash injury was the 8th leading cause of death in the world (WHO, 2018). The numbers of roadway crash fatalities in the United States were 37,133 and 40,000 in 2017 and 2018, respectively (Highway Traffic Safety Administration and Department of Transportation, 2017; U.S. Department of Transportation-NHTSA, 2018). These statistics have made roadway safety a critical concern. AVs are considered an effective solution to significantly enhance traffic safety, as they reduce or even eliminate drivers which contribute to 94% of the crashes in the U.S. (Gora and Rüb, 2016; Morando et al., 2018; Wayomo, 2018).
On the other hand, there are traffic operation obstacles that deteriorate roadway performance. Among different roadway components, urban arterials experience traffic congestion on a daily basis, as they connect major activity centers, and carry high traffic volumes. Moreover, urban arterials are associated with closely spaced signalized and unsignalized intersections; among which, unsignalized intersections are more complicated, as driving behaviors are entirely dependent upon the drivers’ judgment. This high traffic congestion and complexity not only diminishes traffic operation, but also results in higher exposure, and consequently, lower traffic safety (American Association of State Highway and Transportation Officials (AASHTO), 2011; Federal Highway Administration (FHWA), 2010; Khan et al., 2017). Hence, it is important to analyze how AVs perform in urban arterials nearby unsignalized intersections or driveways.
Due to a lack of real-world data, the operational and safety effects of AVs are mainly determined through implementing micro-simulation software packages. VISSIM was used to assess how different levels of AV MPR, and traffic congestion, affect traffic safety and operational performance nearby a driveway on an urban arterial (Mousavi et al., 2021). A total of 24 scenarios were developed, considering four levels of traffic level of service (LOS); including LOS A, LOS B, LOS C, LOS D, and six levels of AV MPRs; 0%, 10%, 25%, 50%, 75%, and 100%.

Traffic operation was analyzed using two different performance measures; one, traffic density; and two, traffic speed. The analysis was done for each combination of traffic LOS and AV MPR, and each traffic lane separately. The results indicated that autonomous vehicles have the capability to improve traffic operation by reducing the overall traffic density, especially for higher traffic LOS (including LOS C and LOS D). At LOS D, in the fully AV environment, high-density points could be observed along the lanes, due to the fact that the AVs are not connected. In other words, since the AVs do not communicate, the arterial vehicles do not get notified when a driveway vehicle intends to merge. Hence, the density increased in some situations where there was not enough gap. Moreover, analyzing the average operating speed of the network indicated that at the same LOS, any increase in the AV MPR resulted in an increase in the average speed of the network. Hence, AVs provide a smoother traffic flow and consequently, a higher average speed.
REFERENCES
Ajitha, T., Vanajakshi, L., Subramanian, S.C., 2015. Real-time traffic density estimation without reliable side road data. J. Comput. Civ. Eng. 29 2 , 1–8. doi:10.1061/(ASCE)CP.1943-5487.0000310
American Association of State Highway and Transportation Officials (AASHTO), 2011. A Policy on Geometric Design of Highways and Streets.
Federal Highway Administration (FHWA), 2010. Access Management in the Vicinity of Intersections. Department of Transportation.
Gora, P., Rüb, I., 2016. Traffic Models for Self-driving Connected Cars, in: Transportation Research Procedia. Elsevier B.V., pp. 2207–2216. doi:10.1016/j.trpro.2016.05.236
Highway Traffic Safety Administration, N., Department of Transportation, U., 2017. Research Note: 2017 Fatal Motor Vehicle Crashes: Overview October .
Khan, S.M., Mitchell, J., Chowdhury, M., Dey, K., Huynh, N., 2017. Operational analysis of a connected vehicle-supported access control on urban arterials. IET Intell. Transp. Syst. 12.
Morando, M.M., Tian, Q., Truong, L.T., Vu, H.L., 2018. Studying the Safety Impact of Autonomous Vehicles Using Simulation-Based Surrogate Safety Measures. J. Adv. Transp. doi:10.1155/2018/6135183
Mousavi, S.M., Osman, O.A., Lord, D., Dixon, K.K., Dadashova, B., 2021. Investigating the safety and operational benefits of mixed traffic environments with different automated vehicle market penetration rates in the proximity of a driveway on an urban arterial. Accid. Anal. Prev. 152, 105982. doi:10.1016/j.aap.2021.105982
U.S. Department of Transportation-NHTSA, 2018. Fatal Motor Vehicle Crashes 2017: Overview. Dot Hs 812603 October , 1–9.
Waymo, 2018. On the Road to Fully Self-Driving.
WHO, 2018. Global Status Report on Road Safety 2018: Summary.
Comments