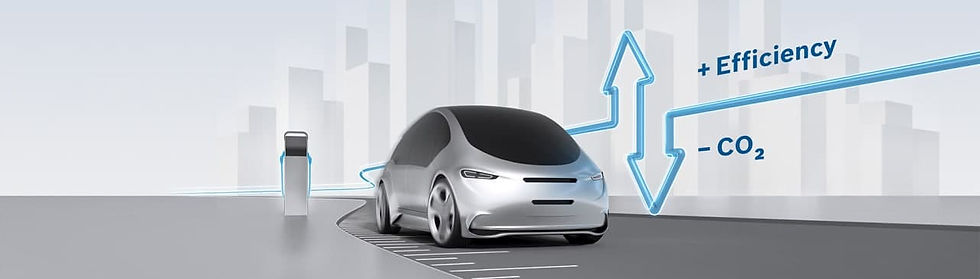
Image Credits: https://www.bosch-mobility-solutions.com/en/solutions/powertrain/battery-electric/electric-drive/
The past decade has seen the rapid development of electrified vehicles. To manage the use of energy sources in an electrified drivetrain, a supervisory controller is essential. An Energy Management Strategy (EMS) distributes power between the energy sources in an electrified vehicle while meeting the driver’s power demand. Monitoring power consumption is critical for the different aspects of electrified vehicles; from minimizing the fuel/electricity purchase, to the vehicle's overall performance.
Various EMSs have been proposed for the application of electrified vehicles, and are generally categorized into methods based on rules and logic, or the approaches used to integrate optimization tools. Rule-based methods are real-time implementable, easy to understand, and suitable for commercial applications. Although EMSs are the Original Equipment Manufacturers (OEMs) confidential documents, assessing the data recorded during tests of the electrified vehicles has shown that OEMs have widely deployed rule-based EMSs for their vehicles. These rules are developed based on engineering expertise and best practices and may deviate the EMS’s performance from optimal levels. Additionally, rule-based control methods cannot be easily modified for a different powertrain architecture. To further improve the performance of rule-based controllers', research efforts have been directed towards incorporating optimization-based methods to rule-based approaches in order to maximize the EMS controller’s capability. Optimization-based algorithms distribute the power, along with the drivetrain, in an optimal way. This optimal distribution requires having complete knowledge of the drive cycle beforehand, partially accessing the future drive mission, or the real-time drive cycle information. To enable the real-time capability of the controller, methods that require complete knowledge of the drive cycle would not be reasonable for actual in-vehicle implementation. Prediction-based optimization algorithms, however, show outstanding performance, embedding the future drive cycle profile to the controller. Although optimization-based algorithms provide profound performance, their implementation can be very challenging for OEMs due to the high computational resources, real-time implementation issues, and the optimization method's complexity. Consequently, the vehicle industry has not completely welcomed prediction-based methods due to reliability issues with the predicted parameters'.
With the rapid advancement of Intelligent Transportation Systems (ITS) and autonomous vehicles' progress, the accessibility of traffic data, road conditions, and vehicle information is more feasible, which renders prediction-based algorithms a viable strategy for the future of electrified vehicle embedded controllers.
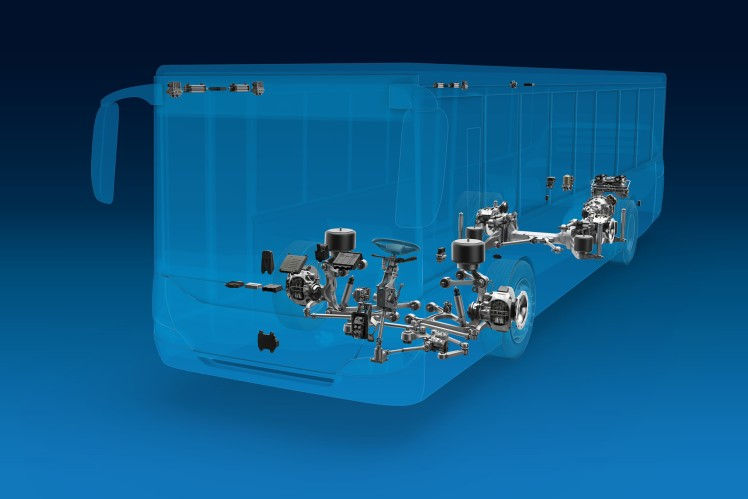
Image Credits: https://press.zf.com/press/en/media/media_26624.html
V2X communication systems offer valuable knowledge on charging station locations, traffic lights, traffic congestion, and route distance information in order to plan the power distribution for the electrified drivetrain more efficiently. This information is integrated into optimization-based algorithms, which enhances prediction accuracy and reliability. Another benefit of V2X communication systems for the EMSs is their capacity to utilize connected vehicles and cloud-based EMS controllers, thus reducing the need for high computational resources.
Data availability through V2X and ITS has also paved the way for the enablement of machine-learning-based EMSs. Critical parameters that affect the power distribution can be easily integrated into machine learning-based EMSs. Examples of these parameters include the trip distances, driver’s behavior, and road geometry. Although machine learning algorithms may require a good deal of offline training that can be time-consuming, they are implementable in real-time. The quality of offline training data will therefore directly impact the real-time EMS controller performance. At the offline stage, optimization-based algorithms can be utilized to prepare a training dataset that enables the machine learning EMS controllers to have a near-optimal performance.
In summary, the EMS control system plays a vital role in the development of an electrified vehicle. Its main task is to distribute power along with the available energy sources in an electrified vehicle. The goal is to design an EMS that optimally divides power, with low computational resources. The objective optimization may vary for the application of electrified vehicles. For a pure electric vehicle (PEVs), the goal can be optimal battery performance in terms of charge/discharge current. For a plug-in hybrid electric vehicle (PHEV), the aim of optimal EMS can be minimizing fuel consumption. Advances in data-driven methods promise the integration of intelligent control methods for EMSs purposes, especially through the introduction of autonomous vehicles. In the future, challenges in EMSs implementation and application-specific EMSs can be elaborated.
Comments